In a time of storms: Mapping crucial coastal zones with advanced bathymetric imaging
Lidar and artificial intelligence help us understand the current state and eventual destiny of our coastlines
The skies may have cleared, but the extent of destruction wrought by 2021’s Hurricane Ida has not been completely tallied. In many ways, it may foretell the future of storms in the Gulf of Mexico in terms of meteorology, geography, and oceanography, all shaped by climate change, it may represent a new level of “perfect storm.”
As it approached the complex Louisiana coastline, Ida exhibited a level of “rapid intensification” that surprised experts, passing over deep levels of warm water, which fueled the storm and accelerated its winds. The coast of Louisiana used to consist of thousands of barrier islands and peninsulas that slowed down approaching storms and shielded residents from the worst surge flooding. But in the early twentieth century, dozens of canals were dredged to to allow for the transportation of oil by ship and pipeline. These new canals allowed salt water to push up through rivers and streams. Combined with rising sea levels, the coastline is fundamentally transformed, and now entire neighborhoods, roads, and other structures are vulnerable to even small storm events. Since the 1930s, Louisiana’s coast has lost 1,900 square miles of land, primarily marshes.
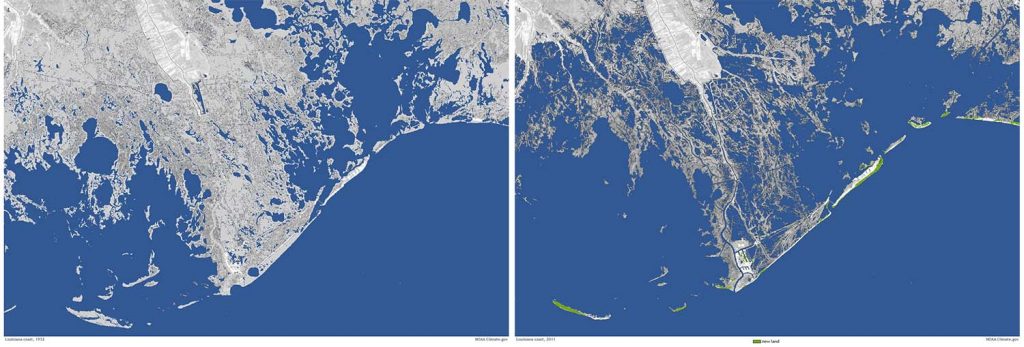
The protective marshland of the coastline has degraded into a web of land, swamp, levees and floodwalls, maintained by local governments and the U.S. Army Corps of Engineers. This leaves the entire area vulnerable to storms and further coastal change when another hurricane arrives.
One tool for understanding this complex and changing environment is the United States Geological Survey (USGS) Coastal Change Forecast model, which is used to estimate the impacts of elevated waves and storm surge along the coast due to extreme storms. This model has been in use since 2011 and is continually improved.
In addition to informing the public, forecasts were used to determine placement of sensors for USGS real-time response to Hurricane Ida. After landfall of a storm, the Coastal Change Hazards team uses NOAA imagery, USGS CoastCams, other beach cams, Digital Elevation Models (DEMs) constructed from post-storm aerial imagery and lidar data, and observations from USGS sensors to validate forecasts.
Understanding the position and height of coastal dunes, waterways, and other landscape features, as well as how they change over time, is critical to the USGS mission of predicting impacts to coastal infrastructure and habitat caused by storms and hurricanes.
Imaging threatened coasts with topo-bathymetry
A key tool for the USGS and similar organizations is airborne lidar topo-bathy systems. These are precision mapping instruments extensively used to map coastal zones and inland waters from land to a depth of 70 meters. These surveys generate a wide range of products, including nautical charts, seamless topo-bathy maps, and benthic habitat classification maps. The Teledyne Optech CZMIL (Coastal Zone Mapping and Imaging Lidar) system is an integrated lidar-imagery sensor system, designed to survey a wide selection of water types, ranging from moderately clear to predominantly turbid. With the optional hyperspectral imaging system and digital metric camera, the CZMIL provides fused lidar and imagery products for a wide range of uses.
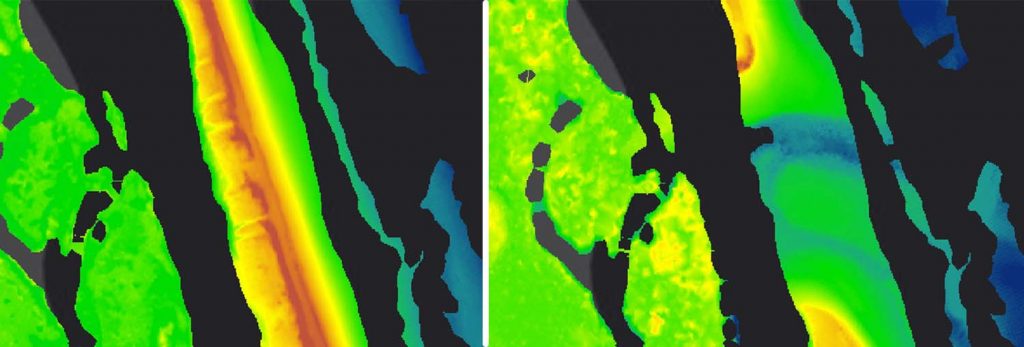
Getting it right
To generate accurate topo-bathy point cloud products, each pulse return from the CZMIL must first be classified as land or water in order to apply the appropriate refraction corrections to the pulse. Typically, airborne lidar topo-bathy systems employ an infrared channel to map the water surface to discriminate between these land and water returns. Low reflective environments like wetlands, however, pose a significant challenge to commonly used statistical-based techniques that primarily exploit infrared waveform return features to classify land and water. This may result in inaccurate land/water classifications and require significant manual correction before the finalized products can be generated. Teledyne Optech investigated state-of-the-art Machine Learning techniques to solve this challenge. In particular, Deep Learning was evaluated to meet and exceed the accuracy of prior techniques, which in turn would result in considerable time and cost savings for the customers.
After significant research efforts, a novel approach using a Recurrent Neural Network (RNN) architecture, based on Long Short-Term Memory (LSTM) was identified. The introduction of LSTM takes advantage of the infrared intensity relationship within the signal time series and is the primary method of classification. In the absence of an infrared return signal, a 1D Visual Geometry Group (VGG) network is applied to the green returns (7 shallow channels) through a convolutional operation. Finally, a pooling operation is applied to incorporate the output of both architectures for the final prediction.
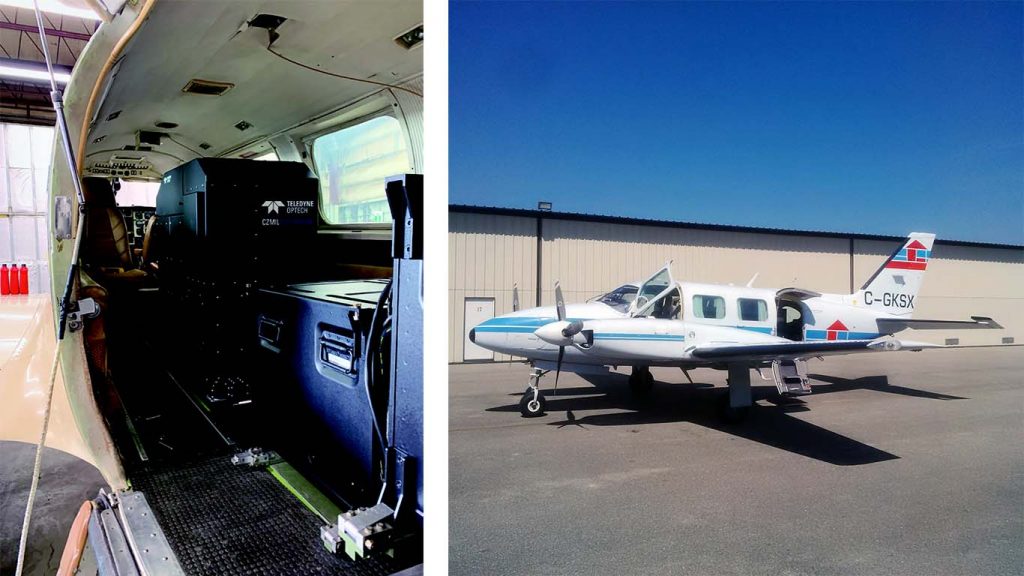
To develop the initial model, a total of 7 CZMIL Nova datasets, acquired over a variety of coastal environments, were used for training and testing of the model. Training was performed using two well-labelled flight lines containing a total of more than 2 million lidar points and validated using the remaining data.
The initial model achieved better than 95% accuracy, while also producing labelled results easier for an operator to clean up versus the previous rule-based method. In early testing, a reduction of editing time ranging from 25-40% for a typical dataset was observed. This was aided in part by visualizing not just the land/water labels, but also the confidence the AI had in this prediction, shown as a percentage (higher is more confident). This allows users to focus on data points where the classification confidence was low.

Noise: No problem
Another aspect of processing topo-bathy lidar data is the automation of the manual edit.ing process after corrections are applied. This task is a critical part of the data processing workflow to generate noise-free final point cloud products. Based on similar Deep Learning architectures developed for topo lidar and multi-beam sonar systems, Teledyne Optech developed a bathymetric noise classifier using a 3D Convolutional Neural Network (CNN). The noise classifier has a strong ability to learn the feature representation of noise in bathymetric lidar, which allows the automation of the noise editing process by invalidating system noise and water column noise returns.
Going supernova
In early 2021, Teledyne Optech introduced a new generation of the CZMIL mapping system with the CZMIL SuperNova. This new system includes two to three times the data measurement
rate of the previous-generation Nova, as well as an optimized scanning pattern to normalize the point distribution across the swath, resulting in a high-density, more consistently spaced point cloud product. The increased data rate means a corresponding increase in data processing time, and the assistance of the new Land/Water Classifier AI is critical to keeping this time reasonable. To further increase the AI accuracy and efficiency, a trained model that is unique to each system is produced.
This model is trained initially using datasets gathered as the unit is being flight tested and refined over time, even after delivery to the customer, to meet their unique operating requirements. The architecture was also enhanced to use the single deep green channel in place of the 7 shallow channels, for marked improvement in classification accuracy. For the first system produced and delivered to a customer, early metrics indicate an increase in accuracy from 95% to over 99%, which means the end product requires minimal editing by operators. In early testing, the corresponding Noise Classifier for CZMIL Nova is well-suited for SuperNova, offering similar performance. As more of these systems are produced, these AI models will be continuously refined and improved with new data to keep our customers on the leading edge
We see that a targeted application of novel Deep Learning architectures can address specific processing challenges in the topo-bathy lidar processing workflow. This gives us a significant increase in accuracy and reduction in the time taken to produce a noise-free point cloud product. This data product is the critical first step in producing accurate, timely information about the world’s coastal zone environment. We hope that this will feed directly to key decision makers, policy advisors and more to monitor and manage these increasingly threatened natural environments.
A very stormy outlook
Ironically, Ida made landfall exactly 16 years to the day after Hurricane Katrina, which was the costliest storm in U.S. history, which also devastated Louisiana back in 2005. That storm was considered a “100-year hurricane,” a term that is becoming less and less believable every year. Thanks to Ida, the Gulf Coast has had a category 4 or 5 landfall each year for the last four years.
The past few years have seen a series of storms that were also driven by the same rapid intensification phenomenon as Ida: Hurricane Irma hitting the Florida Keys in 2017, Michael blowing over the Florida Panhandle in 2018, and then Hurricane Laura devastating Lake Charles, Louisiana in 2020.
For the foreseeable future, living near the Gulf Coasts will mean living with the risk of hurricanes and severe flooding. Researchers will be working furiously to understand how the changing coastline is driving this intensification and what we can do about it. Solutions today range from to huge manmade structures to restocking historical oyster shoals.
As organizations like NOAA and the USGS have shown, the data that drives these solutions will certainly come from advanced lidar bathymetry.