SWIR Imaging for Apples, part II: The Multispectral Future of Food Inspection
The limitations of any one spectrum mean that the future may lie in image fusion and AI-powered algorithms.
As we covered in part I, Short-Wave Infrared (SWIR) imaging reveals extraordinarily valuable information on the status and value of complex fresh foods, like apples – information that the visible spectrum cannot provide. But alone, SWIR also has limitations, as well. So where do we go from here?
The value of optical imaging for non-destructive food inspection and grading means that this a continual focus for R&D. The automatic detection of “product quality” in food remains a significant challenge. The detection of physical variables, across curved surfaces, the discrimination between defects and natural features, invisible and subsurface defects, depends on the reliability of underlying algorithms, and the speed and performance of rapid optical detection systems. These challenges can reduce the accuracy of inspection, increase costs, or slow a production line.
Today we see the use of monochrome, color(VIS), ultraviolet (UV), near-infrared(NIR), short-wave infrared(SWIR), and hyperspectral imaging systems all being used to sort our food. Looking at SWIR imaging methods, it’s almost impossible to simultaneously provide spatial and spectral information on physical and chemical properties of whole objects. And those are the qualities we want to know. For example, a spectrometric system can provide the nondestructive assessment of the soluble solids content (SSC) of fruits. This is a specific measurement that has been shown to be a powerful tool for predicting the internal qualities of fruits. But spectroscopic measurement only collects information from a single area on a sample at a time, limiting its use for surface defect detection on the whole fruit.
Like any inspection industry, the pressure is always to go faster, with higher quality output, at a lower cost. Once solution is hyperspectral imaging technology can integrate the characteristics of both spectroscopy and imaging into one system, providing heterogeneous information reflecting multiple food quality features which predict the salability and longevity of fresh produce.
Using Hyperspectral Imaging to See More at Once
And people are picky about apples. Taste is just the beginning. In Asian countries, a premium apple can be used as a gift for special ceremonies, such as Lunar New Year cerebrations. Here, this means a large size, bright red skin color, and high sugar content.
Hyperspectral imaging is one approach that can give us valuable information on all these properties simultaneously, from the size and shape, colour and even the chemical composition of the surfaces being observed. The traditional use of the hyperspectral imaging techniques has been limited almost exclusively to the spectral region of the visible(380-740nm) and the VNIR (750-1000nm) accessible with silicon-based array detectors.
Fruit size is one of the most important factors used to determine the value of the fruit, and larger fruits are more expensive than smaller ones. To produce larger fruits, a range of cultivation techniques are applied. This can include physical methods, like pruning and thinning, but also chemical methods like plant growth regulators. But the use (or over-use) of these treatments can have side effects, such as fruit elongation, which is not preferred in countries like Japan, China, and Korea, where people prefer round-shaped apple fruits like the Fuji apple or Asian pear. How much is too much? For which market? You need to measure both the treatment and the outcome to know what’s going to work for your system over time.

However, the development of real-time inspection based on hyperspectral imaging has encountered bottlenecks. Hyperspectral images are those where each pixel forms an almost continuous spectrum, with each spatial location of a hyperspectral image containing hundreds of bands. This results in huge data sets that increase the acquisition and processing requirements of the entire inspection system and often excluding the possibility (or practicality) of a real-time application.
Multispectral Imaging: A More Focused Option
Another solution is multispectral imaging. Very similar to hyperspectral imaging, multispectral still allows you to obtain ultraviolet, visible, and infrared spectra imaging for each test sample. But instead of capturing everything across a continuous band, you’re capturing image data within specifically chosen, discontinuous spectral ranges. For example, a typical multispectral imaging system can give an image with generally three to 20 bands across a larger range, such as two from UV, three from VIS, and five from IR.
This allows you select narrow-band images over a discrete spectral range, generating feature-specific wavelengths of each pixel in a targeted object, drastically reducing the bandwidth you need. This also allows the speeds required to scan large amounts of product quickly, meeting the needs of the industry for rapid identification and detection.

Multispectral imaging systems can be developed based on filtering and dispersing devices (such as optical and tunable filters). With smaller, simpler systems that can work much more quickly, multispectral imaging offers a lot of advantages. But, you have to know exactly what you’re looking for. You need to build your sensor and system around the very specific information that will yield the most decisive output. This becomes the most challenging task for multispectral imaging, selecting wavelengths to represent the specific features that characterize interested objects.
Regarding food quality and safety analyses, the most commonly used multispectral imaging systems are designed to acquire spectral data in the VISNIR region (380 to 2500 nm). The collected spectra can provide complex structural features of samples associated with strong absorptions of pigments and overtones (such as first overtone, second overtone, and third overtone), or combination modes (deformation and stretching) of molecular bonds (such as C–H (aliphatic and aromatic), C–O (carboxyl), N–H (amide and amine), and O–H (hydroxyl) functional groups).
Multispectral images can obtain 3-dimensional data that can be selectively transformed into 2D chemical images via multivariate modeling. This approach allows you to predict and visualize quality parameters of fruits and vegetables:
- Physical: texture, water-holding capacity
- Chemical: starch and protein content
- Microbiological: viral and fungal content
- Adulteration: species, origin
- Gradation: ripeness, storage period
- Contamination: forchlorfenuron, imidacloprid
- Defects: bruises, decay
Such quality parameters of plant foods can not only be qualitatively or quantitatively determined, but they can also be spatially visualized, which enables multispectral imaging to realize nondestructive and nonhomogeneous quality measurements.
For example, if we can’t directly measure SSC with spectroscopy, we can instead indirectly measure it with multispectral imaging tools. Other research has confirmed how specific wavelength absorption corresponds to the SSC or sugar content of fruits:
- The 535 and 680 nm bands are related to major pigments (anthocyanin and chlorophyll) in apples, and these pigments of apples will change with change of its ripeness.
- 760 nm correlates with water content, which is inversely proportional to SSC in fruits.
- Absorption at 730 and 900 nm are related to carbohydrates which can determine the concentration of sugar solutions
- Around 900 nm has been shown to correspond to SSC of multiple fruit species (apple, pear, and peach)
Another study found that many points on the VIS-NIR spectrum (400–5000 nm) could be useful for detecting something as specific as bruises in an apple, created one hour before the experiment. When combining these imaging ranges, with different noise and performance characteristics, it’s necessary to perform more advanced image analysis to gain an accurate result. One common technique is Minimum Noise Fraction (MNF) transform, an algorithm that includes two consecutive data reduction operations. The first is based on an estimation of noise in the data as represented by a correlation matrix. This transformation then decorrelates and rescales the noise by variance. Then second transform considers the information between band noise, giving weighted information about the variance across all bands in the raw data set. This kind of analyses make it possible to distinguish between areas with defects in the tissue and the sound ones.

Too much multispectral data? Bring in the AI
Even though there is data streamlining by going with multispectral instead of a hyperspectral imaging solution, that doesn’t mean that it’s actually simple. There are still many relevant bandwidths to consider. Research has shown that some bands have correlational value. This can be very helpful when we’re using data to infer another unmeasured quality (such as SSC described earlier).
How complicated can it get? To measure strawberry firmness alone, you can use 19 discontinuous wavelengths (405, 435, 450, 470, 505, 525, 570, 590, 630, 645, 660, 700, 780, 850, 870, 890, 910, 940, and 970 nm)!
While researchers were already applying neural networks to food quality inspection back in 2002
Like anything related to practical applications of machine learning and artificial intelligence, the field is changing quickly. Multiple research teams are looking at combining multispectral datasets with different neural network learning approaches, generating accuracy results that are as good, if not better than state-of-the-art real-time processing.
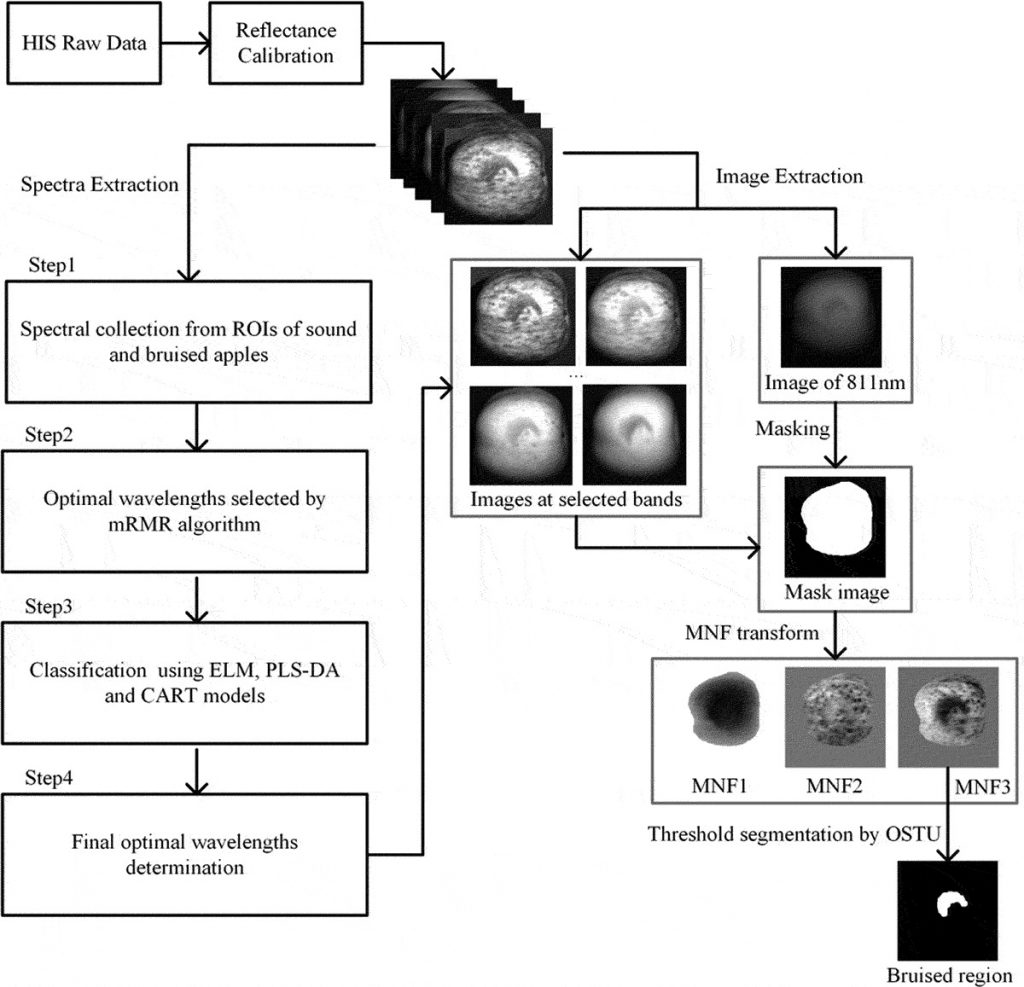
Neural networks will keep getting smarter. Common algorithms such as backpropagation mean that the networks can learn on their own, outpacing what we can teach them with limited data sets. Look forward to a time in the future where food inspection systems will be able to quickly produce almost flawlessly accurate results, and we won’t know exactly how their doing it!
Teledyne DALSA Tech in Food Production
Teledyne DALSA’s SWIR GigE line scan cameras feature a cutting-edge InGaAs sensor in a compact package for a wide variety of machine vision applications.
This high speed, high resolution camera is the first product in DALSA’s SWIR family. Linea SWIR features a cutting-edge InGaAs sensor in a compact package that is suitable for a wide variety of applications. With exceptional responsivity and low noise, this camera allows customers to see their products like never before. Linea SWIR is available as a 1k resolution camera with highly responsive 12.5 µm pixels, or a 512-resolution camera with larger 25 µm pixels.
Like all Linea models, Linea SWIR shares the robust build quality and advanced functionality that is part of Teledyne DALSA’s unmatched line scan heritage. Linea SWIR is packed with features to make your machine vision job easier, including high speed, high sensitivity, cycling mode, and programmable I/Os. The versatile Linea SWIR is ideal for applications such as optical sorting, solar panel inspection, and general purpose machine vision.