Embedded Vision in 2019
The future of embedded vision includes lower costs and more artificial intelligence inside
New imaging applications are booming, from collaborative robots, to drones fighting fires or monitoring farms, to biometric face recognition, and point-of-care hand-held medical devices at home. Established machine vision applications for manufacturing are also being remade: initiatives like Germany’s Industry 4.0 and Made in China 2025 were formulated to keep entire national industrial sectors competitive by introducing ‘smarter’ manufacturing and production – less centralized, more responsive, and more automated. And robots. At automotive OEMs, industrial robots are welding and painting cars. Within warehouses, they are stocking shelves, selecting items for orders, moving goods from docks to trucks, and packaging them for transport. At semiconductor plants, robots move delicate wafers without damaging components. Elsewhere, they’re assisting with gluing, assembling, cutting, grinding, and other routine yet critical tasks. Robotic manufacturers across the automotive, electronic, food packaging and energy sectors are creating a full spectrum of solutions and integrated technologies.
The growing prevalence of these robots is not surprising, because industrial operations, both mundane and complex, are increasingly automated. Cost is a factor in their rise, since the average selling price for a robot has fallen by more than half over the past 30 years, while in key manufacturing countries like China, wages have gone up. Emerging markets have an additional imperative for automation: the need to improve product quality to compete effectively in the export market.
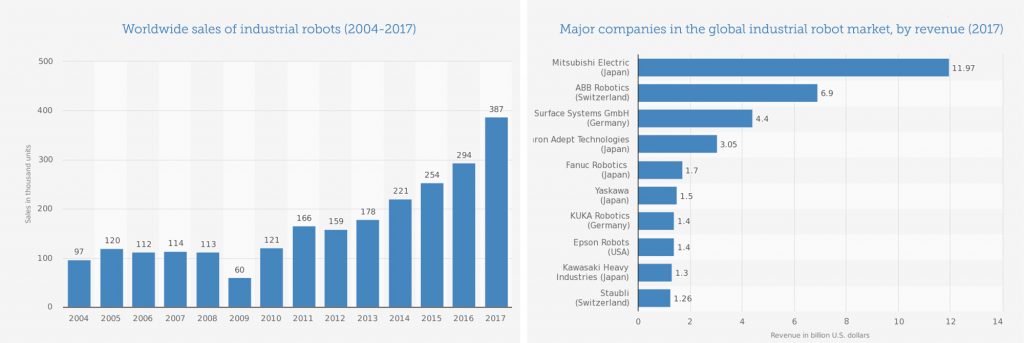
Smaller = More Opportunities
A key enabler of these lower costs has been accessibility. For manufacturing OEMs, system integrators and camera manufacturers, machine vision is typically driven by a consistent set of priorities: size, weight, energy consumption, and unit cost. This is truer for embedded vision applications, where the imaging and processing are combined in the same device, instead of the historically separated camera and PC. Embedded vision means that everything extraneous is typically cut to meet requirements that are measured in milliwatts, millimeters, and fractions of a cent. Providing solutions that can deliver the performance that these new high-growth applications demand within the constraints of embedded vision is critically important to companies in the imaging industry.

The image sensor itself is a significant factor in this pursuit, and care is needed in the choice of the image sensor that will optimize the overall embedded vision system performance. The right image sensors will offer more freedom for an embedded vision designer to reduce not only the bill-of-material but also the footprint of both illumination and optics. To meet market-acceptable price points, sensor manufacturers keep shrinking image sensor pixels—and even the sensors themselves. This cuts their costs because they can fit more chips on a wafer, but it also allows for cost-savings that help to reduce the rest of the system costs, including lower overall power requirements and lower-cost optics.
Putting Vision Processing Inside
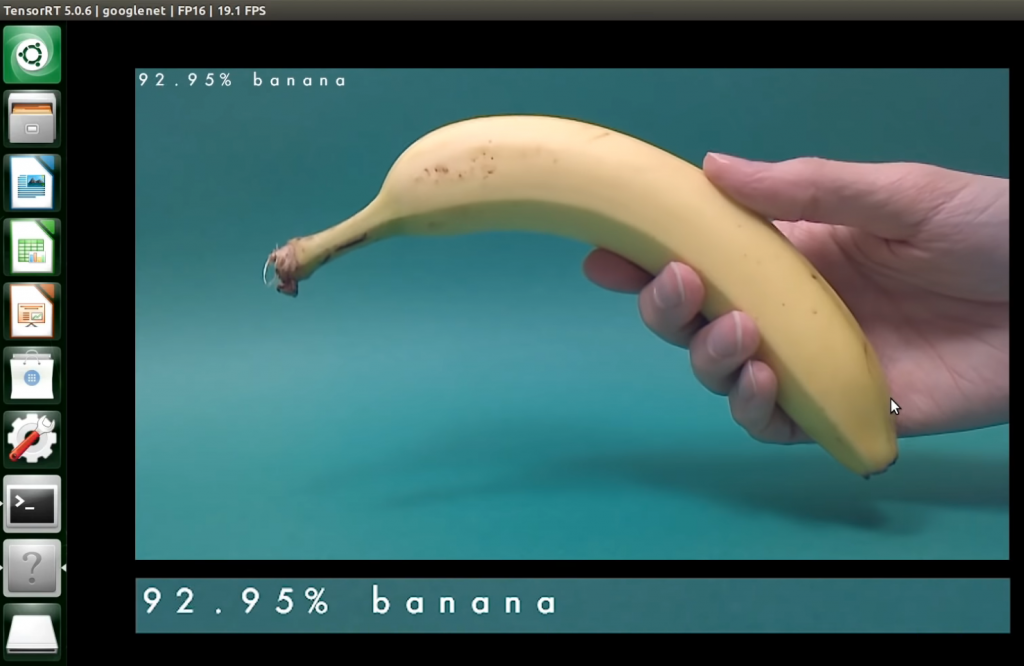
A second change fueling the growth of embedded vision systems is the possibility of including machine learning. Neural networks can be trained in the lab and then loaded directly into an embedded vision system so that it can autonomously identify features and make decisions in real-time. Affordable hardware components developed for the consumer market have drastically reduced the price, size, and requirements for increasingly powerful sensors compared to computers. Single board computers or system on modules, such as the Xilinx Zynq or NVIDIA Jetson pack impressive machine learning capabilities in tiny packages, where image signal processors, such as Qualcomm Snapdragon or Intel Movidius Myriad 2 can bring new levels of image processing to OEM products. At the software level, off-the-shelf software libraries have also made specific vision systems much faster to develop and easier to deploy, even in low quantities.
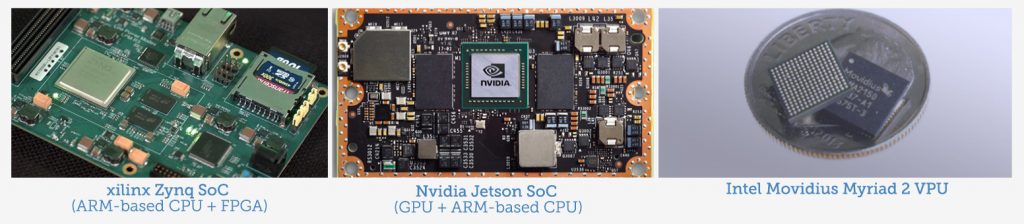
One major way that embedded vision systems differ from PC-based systems is that they’re typically built for specific applications, while PC-based systems are usually intended for general image processing. This is important, as it often increases the complexity of initial integration. However, this complexity is offset by a custom system perfectly suited for the application and for providing return on investment (ROI).
Embedded vision systems are used in applications like self-driving cars, autonomous vehicles in agriculture, digital dermascopes that help specialists make more accurate diagnoses. All of which require performance, but also almost perfect levels of reliability. Today this requires customized solutions with carefully selected hardware, matched to custom software. An even more extreme option would be a customized solution where the image sensor integrating all the processing functions is integrated into a single chip in a 3D stacked fashion to optimize performance and power consumption. However, the cost of developing such a product could be tremendously high, and while a custom sensor it is not totally excluded to reach that level of integration in the long term, today we are at an intermediary step, consisting of embedding particular functions directly into the sensor to reduce computational load and accelerate the processing time.

Where do we go from here? Looking to the future, we can expect new efficiencies and new applications
To guess at the next steps that embedded imaging will take, it’s probably smart to look at another extremely size and power-constrained imaging system that has high demands placed on it: mobile phones. According to Gartner, 80 Percent of Smartphones Shipped Will Have On-Device AI Capabilities by 2022. While many of the expected applications have to do with audio processing, security, and system optimization, several have to do with imaging.
In 2019, mobile phone cameras are already doing a lot of image processing, including color correction, face recognition, imitating lens properties like depth-of-field, HDR, and low-light imaging. Analysts expect the next generation of phone-embedded AI will start knowing more and making more decisions: face identification, content detection (and perhaps censorship), automatically beautified selfies, augmented reality, and even emotion recognition.
While these applications are only tangentially related to most embedded vision applications (outside of security and entertainment), it’s notable that so much effort is going into the computing side of imaging. Camera sensors are starting to hit a limit in how much resolution can be captured with such tiny sensors. The iPhone has had only small changes in sensor size since 2015 and has stuck to the same 12-megapixel resolution. Even with upcoming phone designs sporting three or more cameras, or larger 64-megapixel sensors, it’s expected that all of this data will simply be to better feed the onboard computing resources to create ‘better’ or more useful images at the resolution we’re all seeing already. It’s about getting smarter, instead of higher performance.
Researchers are already looking at what can be accomplished with vision systems that can better understand their environment and make reliable decisions based on that information. Drones that can land themselves. Computationally modelled insect eyes. Autonomous vehicles that can recognize the thin objects that usually challenge lidar, sonar, and stereo camera systems. The PC-to-camera separation will continue to break down, with the possibility of deep neural net models designed specifically for embedded vision systems. Embedded vision opens up entirely new possibilities. We have already seen that it has the potential to disrupt entire industries. Over the next few years, there will be a rapid proliferation of embedded vision technology.